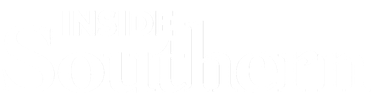
The Early Research Scholars Program, initially designed by the University of California, San Diego (UCSD), is a group-based, team-mentored research experience for students early to midway through the major program. Students will be placed into small groups and then matched with an active research project from the CS department to give students the opportunity to observe the research process in action. Then, students will propose and complete a related research project that they will also present at the end of the program.